高速公路动态交通流Elman神经网络模型 |
| |
引用本文: | 梁新荣, 刘智勇, 毛宗源. 高速公路动态交通流Elman神经网络模型[J]. 交通运输工程学报, 2006, 6(3): 92-96. |
| |
作者姓名: | 梁新荣 刘智勇 毛宗源 |
| |
作者单位: | 1.华南理工大学自动化学院,广东 广州 510640;;2.五邑大学 信息学院,广东 江门 529020 |
| |
摘 要: | 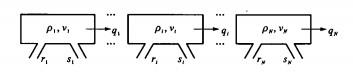 为了提高高速公路交通流建模的精度, 分析了离散的高速公路动态交通流数学模型, 基于Elman网络原理, 建立了回归神经网络交通流模型。回归神经网络的输入层、上下文层、隐含层和输出层的节点数目分别选为8、30、30和2, 采用Levenberg-Marquardt算法对回归神经网络进行训练, 并对一条5路段的高速公路进行仿真。 结果表明: 回归神经网络平均相对误差为8.683 7×10-5, 最大相对误差为4.237 1×10-4, 与BP神经网络和RBF神经网络相比较, Elman回归神经网络能更好地逼近交通流数学模型, 真实地描述交通流基本特性, 能准确地建立动态交通流模型, 适应交通状况的变化。
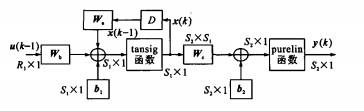
|
关 键 词: | 交通规划 动态交通流 回归神经网络 建模 比较 |
文章编号: | 1671-1637(2006)03-0092-05 |
收稿时间: | 2005-12-06 |
修稿时间: | 2005-12-06 |
本文献已被 CNKI 万方数据 等数据库收录! |
| 点击此处可从《交通运输工程学报》浏览原始摘要信息 |
|
点击此处可从《交通运输工程学报》下载全文 |
|