基于非线性主成分分析和GA-RBF的高速公路交通量预测方法 |
| |
引用本文: | 雷定猷, 马强, 徐新平, 刘青意. 基于非线性主成分分析和GA-RBF的高速公路交通量预测方法[J]. 交通运输工程学报, 2018, 18(3): 210-217. doi: 10.19818/j.cnki.1671-1637.2018.03.021 |
| |
作者姓名: | 雷定猷 马强 徐新平 刘青意 |
| |
作者单位: | 中南大学 交通运输工程学院, 湖南 长沙 410075 |
| |
基金项目: | 国家自然科学基金项目71771218国家自然科学基金项目71771218, 71501190 |
| |
摘 要: | 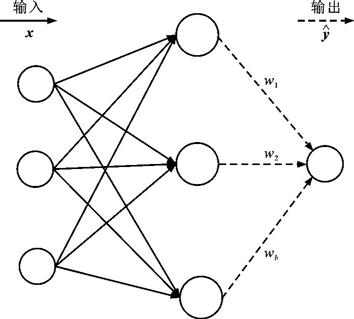 为了提高高速公路交通量的预测精度, 综合考虑高速公路交通量的高度非线性和受多因素影响的特征, 提出一种基于非线性主成分分析和GA-RBF神经网络(NPCA-GA-RBF) 的高速公路交通量预测方法; 确定了高速公路交通量的主要影响指标, 运用非线性主成分分析法降低高速公路交通量影响指标的维数及其相关性, 用少数主成分代替原有的多指标, 以简化神经网络结构; 利用GA优化RBF神经网络的参数, 进一步提高交通量的预测精度; 以普洱市某高速公路为例, 对交通量预测方法进行实例验证。分析结果表明: 2组试验GA-RBF和NPCA-GA-RBF方法的平均相对误差分别比RBF方法降低1.62%、3.53%和2.27%、3.32%, 说明GA优化RBF神经网络能提高RBF方法的交通量预测精度; 与GA-RBF方法相比, 2组试验NPCA-GA-RBF方法的平均相对误差分别降低了1.91%、1.05%, 其交通量预测值更接近实际交通量, 预测结果更为可靠, 表明非线性主成分分析法消除了指标的相关性, 进一步提高了交通量预测精度, 减少了交通量预测复杂度。 可见, NPCA-GA-RBF方法具有更高的交通量预测精度, 能为高速公路的良好管理提供可靠的决策依据, 满足高速公路合理运营管理的客观需求。
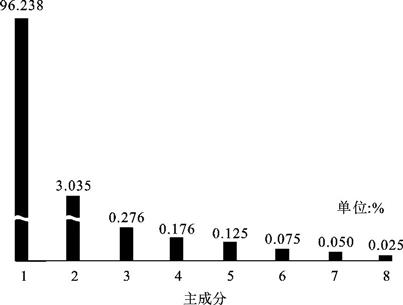
|
关 键 词: | 交通量 预测方法 高速公路 RBF神经网络 非线性主成分分析 遗传算法 |
收稿时间: | 2017-12-12 |
本文献已被 CNKI 等数据库收录! |
| 点击此处可从《交通运输工程学报》浏览原始摘要信息 |
|
点击此处可从《交通运输工程学报》下载免费的PDF全文 |
|