基于车辆行驶轨迹的道路不良驾驶行为实时辨识方法 |
| |
引用本文: | 陆键, 王可, 蒋愚明. 基于车辆行驶轨迹的道路不良驾驶行为实时辨识方法[J]. 交通运输工程学报, 2020, 20(6): 227-235. doi: 10.19818/j.cnki.1671-1637.2020.06.020 |
| |
作者姓名: | 陆键 王可 蒋愚明 |
| |
作者单位: | 同济大学 交通运输工程学院 上海 201804 |
| |
基金项目: | 国家自然科学基金;国家重点研发计划 |
| |
摘 要: | 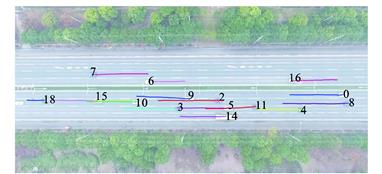 为了提高道路交通安全主动防控能力,以小汽车行驶轨迹数据为研究对象,研究了不良驾驶行为的实时辨识问题;基于无人机拍摄交通流视频提取海量车辆行驶轨迹数据;提出了应用风险度量方法量化典型不良驾驶行为的理论;使用大样本统计分布方法确定不良驾驶行为的特征参数阈值;建立了结合交通环境信息的不良驾驶行为谱,计算了不良驾驶行为谱特征值...
|
关 键 词: | 交通安全 驾驶行为谱 风险度量 不良驾驶行为辨识 人工智能 不平衡类提升算法 |
收稿时间: | 2020-06-13 |
本文献已被 万方数据 等数据库收录! |
| 点击此处可从《交通运输工程学报》浏览原始摘要信息 |
|
点击此处可从《交通运输工程学报》下载免费的PDF全文 |
|