基于改进低秩矩阵补全的交通量数据缺失值插补方法 |
| |
引用本文: | 陈小波, 陈程, 陈蕾, 韦中杰, 蔡英凤, 周俊杰. 基于改进低秩矩阵补全的交通量数据缺失值插补方法[J]. 交通运输工程学报, 2019, 19(5): 180-190. doi: 10.19818/j.cnki.1671-1637.2019.05.018 |
| |
作者姓名: | 陈小波 陈程 陈蕾 韦中杰 蔡英凤 周俊杰 |
| |
作者单位: | 1.江苏大学 汽车与交通工程学院, 江苏 镇江 212013;;2.南京邮电大学 江苏省大数据安全与智能处理重点实验室, 江苏 南京 210003;;3.奇瑞汽车股份有限公司, 安徽 芜湖 241009 |
| |
基金项目: | 国家自然科学基金项目61773184国家自然科学基金项目51875255国家自然科学基金项目61572241江苏省大数据安全与智能处理重点实验室开放课题BDSIP1802 |
| |
摘 要: | 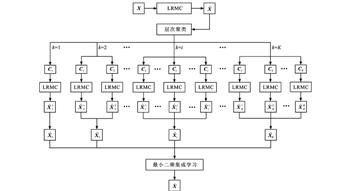 提出了一种低秩矩阵补全的改进方法以研究道路交通量数据缺失值插补问题。应用基于核范数的低秩矩阵补全对交通量数据矩阵中的缺失值进行第1轮插补; 通过层次聚类算法将交通量数据划分为不同类别, 使得同类中的数据具有较强相关性, 异类中的数据具有较弱的相关性; 在每类样本上应用低秩矩阵补全得到缺失值的第2轮插补; 为了减少聚类数的影响, 提出最小二乘回归集成学习方法将不同聚类数下的插补结果进行融合, 得到最终的交通量数据插补结果; 用美国俄勒冈州波特兰市的交通量数据比较了5种方法的插补误差, 并分析了不同聚类数和距离度量方法的影响。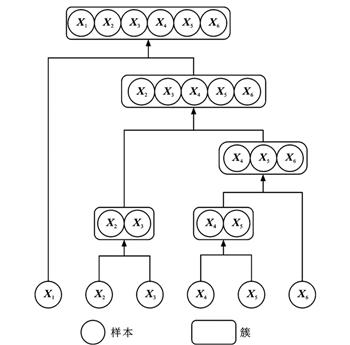 研究结果表明: 在完全随机缺失模式下, 缺失率为10%~60%时, 其相对于传统的低秩矩阵补全模型的插补误差降低了5.93%~9.11%;在随机缺失和混合缺失模式下, 插补误差也分别降低了8.32%~9.55%和8.14%~9.20%;集成不同聚类数下的多个插补结果比单一聚类数下的插补误差降低2.62%~4.76%。可见, 在3种数据缺失模式下, 改进低秩矩阵补全方法降低了交通量数据的插补误差, 能有效提高插补后交通量数据的有效性。
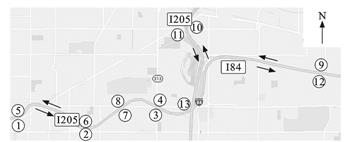
|
关 键 词: | 智能交通 最小二乘回归 缺失值插补 低秩矩阵补全 层次聚类 插补误差 |
收稿时间: | 2019-04-20 |
本文献已被 CNKI 等数据库收录! |
| 点击此处可从《交通运输工程学报》浏览原始摘要信息 |
|
点击此处可从《交通运输工程学报》下载全文 |
|